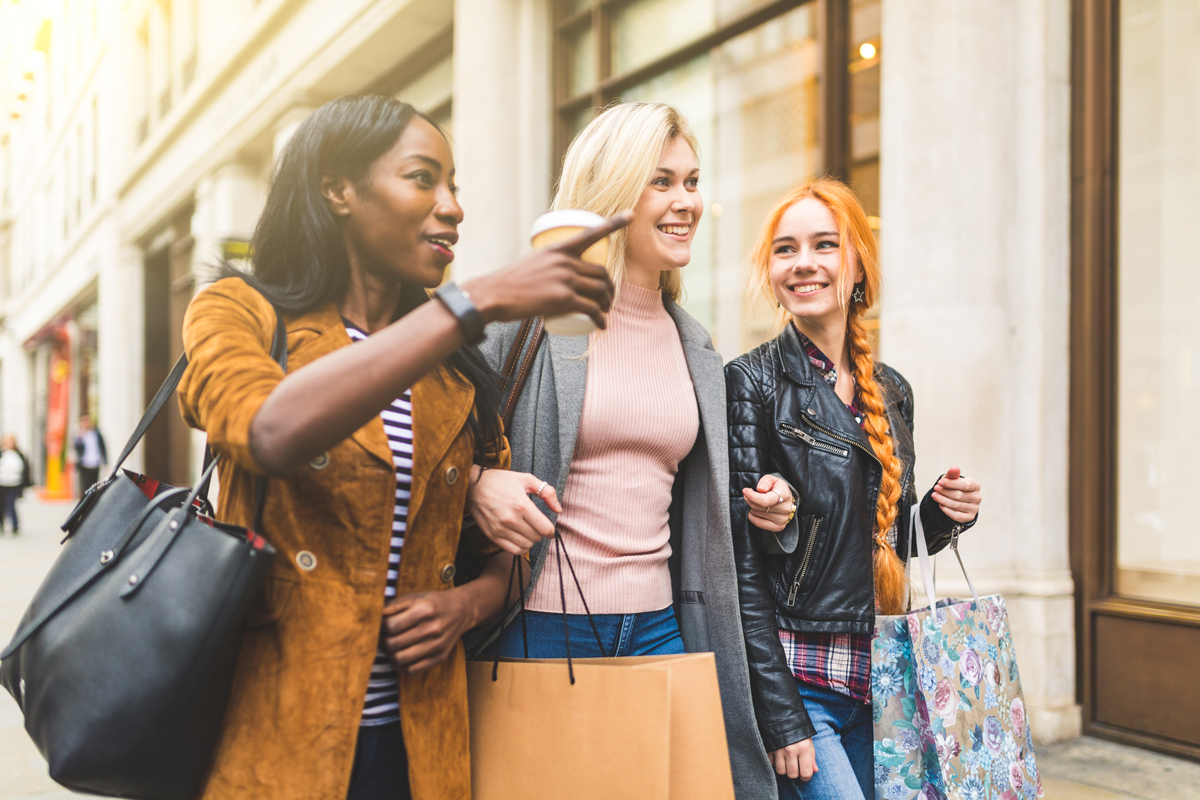
So What… Now What
How the AI/ML Tools of Today are Changing the Game in Customer Experience – if Implemented Correctly
by Bill Barnes
Earlier in my career as the Global Voice of the Customer (VOC) leader at Eastman Chemical Company, CEO Earnie Deavenport asked me to give an overview on our Customer Experience results to his Business General Managers. Eastman had recently won the Malcolm Baldrige National Quality award with Category 7 (customer feedback systems), one of our key strengths. Earnie directed me to challenge the GMs to elevate their engagement by using the VOC to drive strategic and operational business changes.
“Take no prisoners” was my attitude to tell it how it was, with a focus on showcasing our organization’s strengths and opportunities. My primary objective was getting GM buy-in to make improving the VOC part of their defined business strategy, with a tie-in to incentive compensation. After finishing my presentation, Harry, one of our GMs, loudly asked:
“So what, Barnes? You just presented us a lot of soft data on how the customers perceive our performance vs. competition. But, I need hard data to run the business. I need to know what to change and if we make the process changes, will it drive revenue and profitability? Most importantly, how do I know this every day for every customer as they all have different and evolving needs?”
I quickly remembered Harry had a paperweight on his desk that said “In God we Trust, All Others Bring Data.” Harry was ready to jump on board if I could show him how to align his disparate data with VOC to help run the business. Twenty years ago, we did not have the analytical tools or expertise to really answer his questions in real time at the level of granularity he needed to run the business. Then, Burke supported me via ad-hoc linkage work, building static models that utilized state-of-the-art analytical techniques. But even these models were hard to interpret and make the right assumptions to enable model predictive accuracy. And just like today, these models were restricted by what data was available and what unit of analysis we could develop.
As I look at the CX business today with new state-of-the-art Artificial Intelligence/Machine Learning analytical tools that can do what Harry asked for, I see that just developing data analysis tools for integrated data is not enough to align with business strategy. Many companies are not focused on, or aware of, the hard part: Implementation. As organizations embrace AI/ML tools, there are number of implementation success factors to consider:
Organizations should go through customer-centric culture changes to break down organizational silos housing data.
-
- Make sure efforts to develop AI/ML models are driven at the corporate level.
- Ensure that data scientists and/or third-party vendors are aligned with business needs on an ongoing basis.
- Celebrate business improvements that occur during implementation.
- Stay abreast of industry best practices, as AI/ML capabilities quickly evolve.
Building predictive models that quantify future customer behavior requires a blend of art and science. Statistical models must have the right assumptions and data to be valid. Some of the art and science components of implementation to consider include the following:
-
- Identify the business problem/question to solve as your first step.
- Access the data available to solve that problem and assess that data’s accuracy.
- Determine what other data could enhance the answer to your question.
- Select the right AI/ML analytical tools to use.
- Design and test the model to ensure the accuracy level and predictive output desired.
- Educate internal users on how to interpret the model output.
- Provide the right level of service and model customization that will be needed as business dynamics change.
Be prepared and willing to let underlying AI/ML models evolve, grow, and continue to learn as new data that is relevant to the marketplace and customer experiences become available.
-
- One model will not be enough to solve today’s unique, complex business problems. Different functions will have different needs and will require deep dives into specific problems, with ongoing day-to-day service needs.
- Business dynamics will quickly change, and models will need to be recast to handle new dynamics.
- Model interpretation will be the gateway for driving action. High engagement will be necessary to ensure model output is acted upon. Disseminating data output to a wider group alone will not guarantee action.
- Safeguards must be in place to ensure incoming data are running as designed as it constantly feeds model output.
Now back to Harry…
Sometimes we look back on our careers and we might say, “if I only knew then what I know now.” Now, I would have loved to have been able to reply to Harry’s So What Barnes question with:
“Harry, let’s discuss what business problems you are trying to solve, give me access to your disparate data, and I will be back with an answer that says Harry, now what are you going to do?”
That would have felt good.
External Note:
Burke now has Geode|AI, a new data fusion and ML/AI solution used by a team of data scientists who can help you manage your data resources to extract insights and provide foresight.

As Senior Vice President at Burke, Inc., Bill Barnes leverages an extensive background in customer experience to help clients design and implement successful CX programs. With a deep understanding of CX, Bill knows that in order to for a program to be successful, one needs to understand the internal cultural issues that inhibit improvement and measure customer centric culture, which is the foundation of this work.
Interested in reading more? Check out Bill’s other articles:
Overcoming Silos through Customer Centricity
As always, you can follow Burke, Inc. on our LinkedIn, Twitter, Facebook and Instagram pages.
Sources:
Feature Image – ©Siarhei – stock.adobe.com